Collaborative filtering
One key development in personalized recommendation systems has been the use of collaborative filtering. This approach involves analyzing the preferences and behaviors of a group of users, and using that data to make recommendations to individual users. For example, if a group of users who have similar interests and tastes have all liked a particular website, the recommendation system might suggest that website to a new user who exhibits similar behavior.
Content-based recommendation systems
Another important development has been the use of content-based recommendation systems. These systems analyze the content of a website or piece of content, and use that information to make recommendations to users. For example, a content-based recommendation system might analyze the text of an article and use the keywords to recommend similar articles to the reader.
In recent years, personalized recommendation systems have also started to incorporate more data sources, such as social media activity and location data. This has allowed them to make even more personalized recommendations, as they can take into account a wider range of factors that might influence a user's preferences.
Overall, personalized website recommendation systems have come a long way in a short amount of time. They have become an essential tool for improving the user experience on the internet, and are likely to continue to evolve and become even more sophisticated in the future.
The current state of personalization systems
Traditionally websites have personalized their user experience with inhouse recommendation systems requiring expensive coding expertise. However, more affordable SaaS solutions are now available as website plugins that are easy to install and they more or less deliver the same types of recommendations.
Rules-based segmentation is one of the original onsite personalization solutions that’s still used today. For example, a very common segment is based on the rule of whether or not a user abandons a cart. If a cart gets abandoned, a rule can be set to offer a recommendation or a discount.
Behavioral recommendations came next, and again it's still going strong. Marketplaces like Amazon track user behavior (what users view or buy) and then when the user clicks a product the website recommends other “Related Items” and/or “Best Sellers in this Category.” Most websites using personalization today still use these user behavior recommendations, including Amazon.
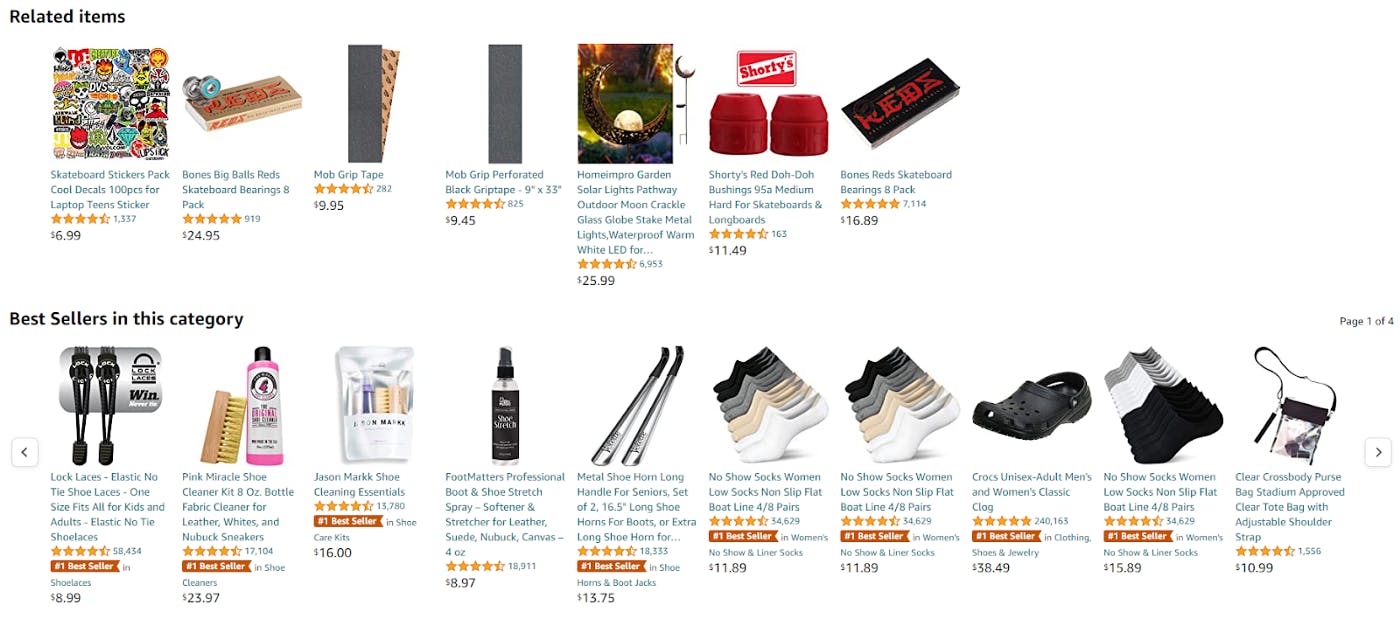
Predictive recommendations are a more recent development, the result of Amazon (and other big companies) refining their algorithms in recent years. Now machine learning can predict what individual visitors want after just a handful of visits to the site. By paying attention to our personal browsing history in real-time, companies with AI-driven recommendations systems can make recommendations to shoppers in real time that are truly 1-to-1. On the Nike website the “You Might Also Like” recommendation box shows items that become more personalized as visitors continue to frequent the site.
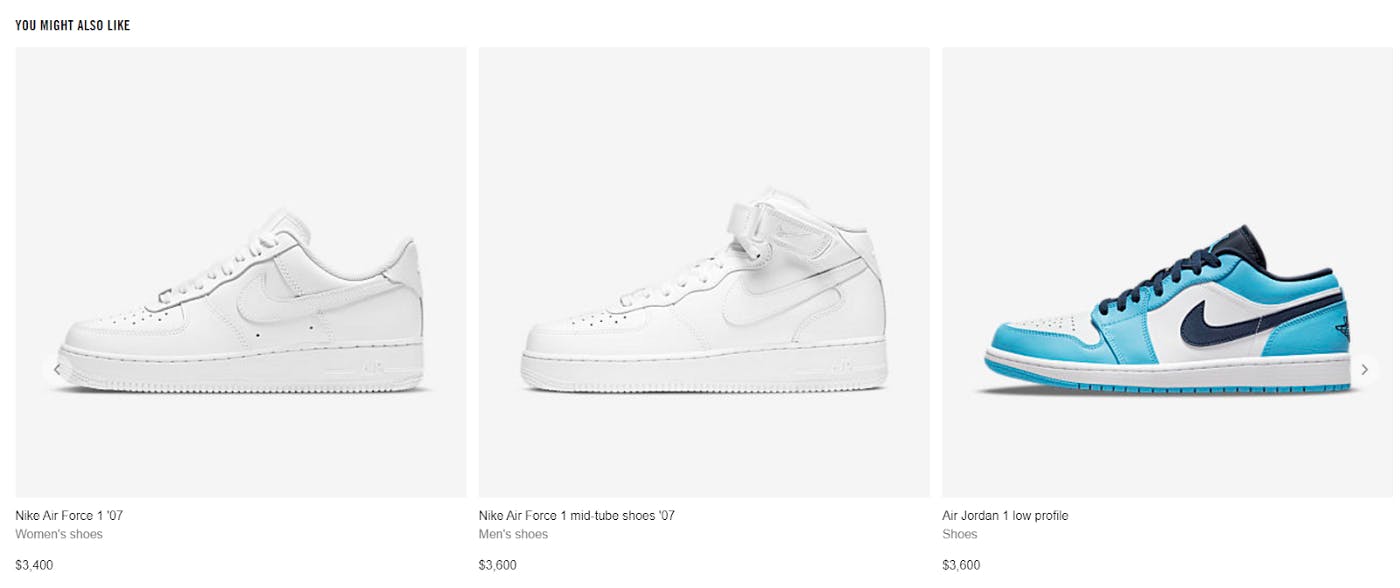
The more the visitor views, adds to cart, or buys, the more accurately the recommendation engine becomes at predicting what that visitor wants to see. It’s at this point that a website begins to make more cross-sells because the products being recommended really resonate with individual shoppers.
The personalized recommendations maturity graph below is an often-referenced standard in ecommerce.
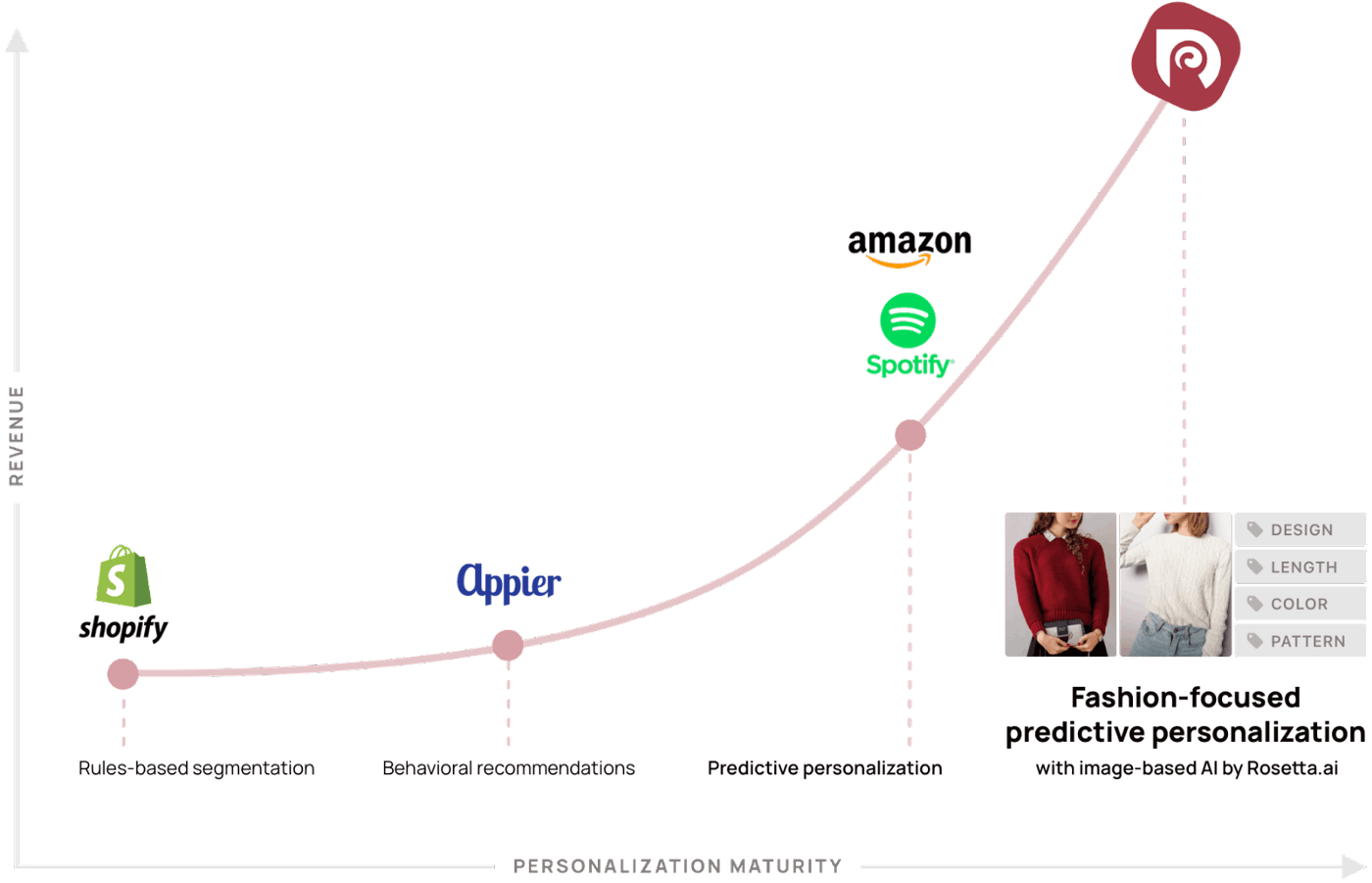
Note that as specialization increases the recommendations get more accurate and deliver more cross-sells and overall revenue.
Drilling down into a specific niche with a tool optimized to do the job better is the next step. In the fashion industry websites and ecommerce platforms use personalized recommendation systems to suggest products and styles to their users. These systems can be tailored to the fashion industry by using data sources and machine learning techniques that are specific to fashion.
For example, a fashion recommendation system might use data on the user's past purchases and browsing history to . It might also analyze the user's social media activity and location data to get a better understanding of their personal style and the types of products they are likely to be interested in.
In addition to using traditional recommendation system techniques, such as collaborative filtering and content-based recommendations, a fashion recommendation system might also use Visual AI image recognition and natural language processing to analyze the user's interactions with the website and thus make more accurate recommendations to the shopper.
Conclusion
Overall, personalized website recommendation systems have come a long way in a short amount of time. They have become an essential tool for improving the user experience on the internet, and are likely to continue to evolve and become even more sophisticated in the future.
Furthermore, a specialized recommendation system for fashion can be a powerful tool for improving the user experience on a fashion website or e-commerce platform, and can help drive sales by suggesting products and styles that are likely to be of interest to the user.